![]() |
Hi, this is Yatao ( |
Research Focus
My research centers on AI capability-driven basic research, structured along two deeply interconnected and synergistic lines of inquiry:- Sci4AI: Advancing AI with Science. AI's progress has long been nurtured by scientific disciplines—for example, the Boltzmann machine rooted in statistical physics. I am dedicated to developing principled AI methodologies grounded in fundamental mathematical and scientific theories, aiming to push the frontiers of AI capabilities.
- AI4Sci: Advancing Science with AI. I am committed to leveraging AI's transformative potential to accelerate discovery and address critical challenges in science and society. My work focuses on developing AI4Sci toolkits that are not only highly accurate and robust but are also increasingly endowed with advanced reasoning capabilities, enabling new modes of scientific inquiry.
Job Openings
Job Openings: I am looking for highly motivated Postdocs, PhDs, vistiting students (e.g., CSC students), interns and RAs. Here is a recent job description (in English) and job description (in Chinese). To gain a better understanding of the PhD program, I highly recommend watching the presentation by Prof Silvija Gradecak-Garaj: Pursuing PhD from a research perspective.◽ Google Form: To help me learn about your aspirations and background, please fill out this Google Form *before* sending an email (to bluewhalelaboratory@gmail.com). This will allow me to give your application the attention it deserves. I sincerely appreciate your understanding and cooperation in this matter.
◽ Prospective Ph.D. students (2026 Spring, Fall): please apply through the NUS Graduate Admission System: https://gradapp.nus.edu.sg/portal/app_manage. More info: https://www.comp.nus.edu.sg/programmes/pg/phdcs/admissions/.
◽ You can follow my rednote(小红书)@bluewhalelab or Twitter X@yataobian to get the latest news/guidelines.
◽I am happy to host (remote) undergraduate/graduate visitors, with the National University of Singapore (NUS) Computer Science.
News
- April 2025: EMPO is released! It represents an early attempt at fully unsupervised incentivization of LLM (R1-Zero-like) reasoning capabilities. This approach requires no supervised information and enhances reasoning by minimizing the predictive entropy of LLMs in a latent semantic space.
- I am invited to serve as an Area Chair of NeurIPS 2025.
- January 2025: Three papers accepted by ICLR 2025. Congrats Qingyang, Hongyi and Yifan! They are about multi-property molecular optimization and AI reliability (eg, preventing harmful concepts generation).
- June 2024: Code released for our ICML 2024 paper: GMT (Graph Multilinear neT)!
- January 2024: Two papers accepted by ICLR 2024. See you at Vienna Austria.
- May 2023: Attend ICLR 2023 at Kigali Rwanda.
- November 2022: Our work of "Learning Neural Set Functions Under the Optimal Subset Oracle" was selected as an oral presentation at NeurIPS 2022 (TOP 0.38%, very low chance!).
- October 2022: I won the Outstanding Mentor Award of Tencent Rhino-Bird Elite Talent Program.
- September 2022: Three papers accepted by NeurIPS 2022. Congrats Zijing, Yongqiang and Zongbo!
- September 2022: I gave a talk at the AI+Chemistry/Chemical Engineering Forum of the 2022 WAIC (World Artificial Intelligence Conference). The topic is about "Data Efficient Molecular Property Prediction via Graph Self-Supervised Pretraining and Optimal Transport Based Finetuning".
- August 2022: We gave a tutorial on Trustworthy Graph Learning at KDD 2022: Trustworthy Graph Learning: Reliability, Explainability, and Privacy Protection.
- June 2022: I am going to give a talk at the DataFunTalk on June 25.
- May 2022: p-Laplacian Based Graph Neural Networks accepted by ICML 2022, congrats Guoji!! pGNN offers a novel message passing scheme derived from the classical graph p-Laplacians, enjoys superior performance on heterophilic graphs, competitive performance on homophilic graphs, and robustness to noisy edges. It is equipped with solid theoretical justifications. Come to check it :-)
- Apr. 2022: Paper on Fine-Tuning Graph Neural Networks via Graph Topology induced Optimal Transport accepted by IJCAI 2022, congrats Jiying! We propose a novel optimal transport-based fine-tuning framework called GTOT-Tuning, it offers efficient knowledge transfer in the pretraining-finetuning framework for learning graph representations.
- Mar. 2022: Drug AI OOD Dataset Curator and Benchmark "DrugOOD" code released, here is the project page.
- Mar. 2022: Neural set function learning paper released: Learning Set Functions Under the Optimal Subset Oracle via Equivariant Variational Inference, here comes the project page. In this paper we present a principled yet practical maximum likelihood learning framework, termed as EquiVSet, that simultaneously meets the following desiderata of learning set functions under the OS oracle: i) permutation invariance of the set mass function; ii) permission of varying ground set; iii) full differentiability; iv) minimum prior; and v) scalability.
- Jan. 2022: "Drug AI OOD Dataset Curator and Benchmark" Paper "DrugOOD" released, here comes the project page. Will be continuously updated.
- Jan. 2022: Two papers accepted by ICLR 2022, one on energy based learning for interpretable ML, the other one on neural docking for protein-protein interaction, congratulations Xinyuan!
- Dec. 2021: Participate in the "Key Issues of AI Ethics and Their Solutions" Symposium (“AI伦理关键问题及其解决路径”学术研讨会) at the Southern University of Science and Technology.
- Nov. 2021: Paper on p-Laplacian Based Graph Neural Networks released.
- Nov. 2021: Paper on Independent SE(3)-Equivariant Models for End-to-End Rigid Protein Docking released.
- Sep. 2021: Paper on GCN robust training method accepted by NeurIPS 2021, congratulations Heng!
- June. 2021: Paper on Energy-based learning for cooperative games released.
- April. 2021: Paper on Self-distilling GNNs accepted by IJCAI 2021, congratulations Yuzhao!
- April. 2021: we gave a tutorial on Advanced Deep Graph Learning at WWW 2021: Advanced Deep Graph Learning: Deeper, Faster, Robuster, and Unsupervised.
- Dec. 2020: paper presentated at NeurIPS2020 on self-supervised graph Transformers for drug discovery.
- Apr. 2019: paper accepted by ICML19.
- Oct. 2018: papers accepted by NeurIPS18 and BNP@NeurIPS18.
- Sep. 2017: paper "Continuous DR-submodular Maximization: Structure and Algorithms" accepted by NeurIPS 2017.
- Since Jun. 2015, I am associated Fellow of the Max Planck ETH Center for Learning Systems.
Blue Whale Lab
Vision and Mission
- I founded the BlueWhaleLab@NUS because I am passionate about the potential of AI to address some of the most pressing challenges in science and society, and I am eager to collaborate with like-minded individuals to achieve this vision.
- The website is launched:https://bluewhale.yataobian.com/
- Why is it called BlueWhaleLab? The blue whale is the largest mammal on Earth, possessing immense potential and capabilities, much like AI with its limitless possibilities. Additionally, the blue whale is one of my favorite animals, with its unique charm and intelligence. It embodies freedom and the pursuit of the stars and the ocean.
- More is coming!
Academic Services
Conference Reviewing/PC/Chair
- Area Chair of NeurIPS 2025, ICLR 2025
- Session Chair of ICLR 2025
- ICML 2025, NeurIPS 2024, ICML 2024, NeurIPS 2023, ICLR 2023, NeurIPS 2022, ICML 2022, ICLR 2022, CVPR 2022, AAAI 2022, AISTATS 2022, NeurIPS 2021, ICCV 2021, CVPR 2021, AAAI 2021, NeurIPS 2020, AAAI 2020, NeurIPS 2019, ICML 2019, AISTATS 2019, STOC 2018, ITCS 2017, NIPS 2016
Journal Reviewing
- Nature, JMLR, T-PAMI
Tutorials @Top Conferences
- Co-tutoring a tutorial on Trustworthy Graph Learning at KDD 2022: Trustworthy Graph Learning: Reliability, Explainability, and Privacy Protection.
- Co-tutoring a tutorial on Advanced Deep Graph Learning at WWW 2021: Advanced Deep Graph Learning: Deeper, Faster, Robuster, and Unsupervised.
Research Experience
Industry Experience
- Google Research
- Tencent AI
Funded Research Projects
- PI@Tencent AI Lab, Rhino-Bird Focused Research Program, 2025/07-2026/07
- PI@Tencent AI Lab, Rhino-Bird Focused Research Program, 2023/07-2024/07
- PI@Tencent AI Lab, Rhino-Bird Focused Research Program, 2022/07-2023/07
- PI@Tencent AI Lab, Rhino-Bird Focused Research Program, 2021/07-2022/07
Selected Papers & Code [Full List]
◽Notice:
My name was also written as (Andrew) An Bian.
Copyright warning:
The copyright of each published paper belongs to the respective publisher.
The local copy is only for non-commercial, personal use.
-
Energy-Based Learning for Cooperative Games, with Applications to Valuation Problems in Machine Learning
ICLR 2022.@inproceedings{bian2022energybased, title={Energy-Based Learning for Cooperative Games, with Applications to Valuation Problems in Machine Learning}, author={Yatao Bian and Yu Rong and Tingyang Xu and Jiaxiang Wu and Andreas Krause and Junzhou Huang}, booktitle={International Conference on Learning Representations}, year={2022}, url={https://openreview.net/forum?id=xLfAgCroImw} }
-
Independent SE(3)-Equivariant Models for End-to-End Rigid Protein Docking
ICLR 2022 Spotlight.@inproceedings{ganea2022independent, title={Independent {SE}(3)-Equivariant Models for End-to-End Rigid Protein Docking}, author={Octavian-Eugen Ganea and Xinyuan Huang and Charlotte Bunne and Yatao Bian and Regina Barzilay and Tommi S. Jaakkola and Andreas Krause}, booktitle={International Conference on Learning Representations}, year={2022}, url={https://openreview.net/forum?id=GQjaI9mLet} }
-
DrugOOD: Out-of-Distribution (OOD) Dataset Curator and Benchmark for AI-aided Drug Discovery--A Focus on Affinity Prediction Problems with Noise Annotations
Preprint 2022.@ARTICLE{2022arXiv220109637J, author = {{Ji}, Yuanfeng and {Zhang}, Lu and {Wu}, Jiaxiang and {Wu}, Bingzhe and {Huang}, Long-Kai and {Xu}, Tingyang and {Rong}, Yu and {Li}, Lanqing and {Ren}, Jie and {Xue}, Ding and {Lai}, Houtim and {Xu}, Shaoyong and {Feng}, Jing and {Liu}, Wei and {Luo}, Ping and {Zhou}, Shuigeng and {Huang}, Junzhou and {Zhao}, Peilin and {Bian}, Yatao}, title = "{DrugOOD: Out-of-Distribution (OOD) Dataset Curator and Benchmark for AI-aided Drug Discovery -- A Focus on Affinity Prediction Problems with Noise Annotations}", journal = {arXiv e-prints}, keywords = {Computer Science - Machine Learning, Computer Science - Artificial Intelligence, Quantitative Biology - Quantitative Methods}, year = 2022, month = jan, eid = {arXiv:2201.09637}, pages = {arXiv:2201.09637}, archivePrefix = {arXiv}, eprint = {2201.09637}, primaryClass = {cs.LG}, adsurl = {https://ui.adsabs.harvard.edu/abs/2022arXiv220109637J}, adsnote = {Provided by the SAO/NASA Astrophysics Data System} }
-
On Self-Distilling Graph Neural Network
IJCAI 2021. -
Graph Information Bottleneck for Subgraph Recognition
ICLR 2021. -
Self-Supervised Graph Transformer on Large-Scale Molecular Data
NeurIPS 2020. -
From Sets to Multisets: Provable Variational Inference for Probabilistic Integer Submodular Models
ICML 2020.@inproceedings{sahin2020sets, title={From Sets to Multisets: Provable Variational Inference for Probabilistic Integer Submodular Models}, author={Sahin, Aytunc and Bian, Yatao and Buhmann, Joachim M and Krause, Andreas}, booktitle={Proceedings of the 37th International Conference on Machine Learning}, year={2020}, publisher={PMLR}, }
-
Provable Non-Convex Optimization and Algorithm Validation via Submodularity
Doctoral thesis, ETH Zurich.@phdthesis{bian2019provable, title={Provable Non-Convex Optimization and Algorithm Validation via Submodularity}, author={Bian, Yatao An}, year={2019}, school={ETH Zurich} }
-
Optimal Continuous DR-Submodular Maximization and Applications to Provable Mean Field Inference
ICML 2019.@inproceedings{bian2019optimalmeanfield, title={Optimal Continuous DR-Submodular Maximization and Applications to Provable Mean Field Inference}, author={Bian, Yatao A. and Buhmann, Joachim M. and Krause, Andreas}, booktitle={Proceedings of the 36th International Conference on Machine Learning}, pages={644--653}, year={2019}, volume={97}, series={Proceedings of Machine Learning Research}, address={Long Beach, California, USA}, month={09--15 Jun}, publisher={PMLR}, }
-
CoLA: Decentralized Linear Learning
NeurIPS 2018.@inproceedings{he2018cola, title={COLA: Communication-Efficient Decentralized Linear Learning}, author={He, Lie and Bian, An and Jaggi, Martin}, booktitle={Advances in Neural Information Processing Systems (NeurIPS)}, pages = {4537--4547}, year={2018} }
-
A Distributed Second-Order Algorithm You Can Trust
ICML 2018.@inproceedings{Celestine2018trust, title={A Distributed Second-Order Algorithm You Can Trust}, author={D{\"u}nner, Celestine and Lucchi, Aurelien and Gargiani, Matilde and Bian, An and Hofmann, Thomas and Jaggi, Martin}, booktitle={ICML}, pages={1357--1365}, year={2018} }
-
Continuous DR-submodular Maximization: Structure and Algorithms
NIPS 2017.@inproceedings{biannips2017nonmonotone, title={Continuous DR-submodular Maximization: Structure and Algorithms}, author={Bian, An and Levy, Kfir Y. and Krause, Andreas and Buhmann, Joachim M.}, booktitle={Advances in Neural Information Processing Systems (NIPS)}, pages={486--496}, year={2017} }
-
Guarantees for Greedy Maximization of Non-submodular Functions with Applications
ICML 2017.@inproceedings{bianicml2017guarantees, title={Guarantees for Greedy Maximization of Non-submodular Functions with Applications}, author={Bian, Andrew An and Buhmann, Joachim M. and Krause, Andreas and Tschiatschek, Sebastian}, booktitle={International Conference on Machine Learning (ICML)}, pages={498--507}, year={2017} }
-
Guaranteed Non-convex Optimization: Submodular Maximization over Continuous Domains
AISTATS 2017.@inproceedings{bian2017guaranteed, title={Guaranteed Non-convex Optimization: Submodular Maximization over Continuous Domains}, author={Bian, Andrew An and Mirzasoleiman, Baharan and Buhmann, Joachim M. and Krause, Andreas}, booktitle={International Conference on Artificial Intelligence and Statistics (AISTATS)}, pages={111--120}, year={2017} }
-
Model Selection for Gaussian Process Regression
GCPR 2017.@inproceedings{gorbach2017model, title={Model Selection for Gaussian Process Regression}, author={Gorbach, Nico S and Bian, Andrew An and Fischer, Benjamin and Bauer, Stefan and Buhmann, Joachim M}, booktitle={German Conference on Pattern Recognition}, pages={306--318}, year={2017} }
-
Information-Theoretic Analysis of MAXCUT Algorithms
ITA 2016.@inproceedings{bian2016information, title={Information-theoretic analysis of MaxCut algorithms}, author={Bian, Yatao and Gronskiy, Alexey and Buhmann, Joachim M}, booktitle={IEEE Information Theory and Applications Workshop (ITA)}, pages={1--5}, url={http://people.inf.ethz.ch/ybian/docs/pa.pdf}, year={2016} }
-
Greedy MAXCUT Algorithms and their Information Content
ITW 2015.@inproceedings{ITW15_BianGB, author = {Yatao Bian and Alexey Gronskiy and Joachim M. Buhmann}, title = {Greedy MaxCut algorithms and their information content}, booktitle = {IEEE Information Theory Workshop (ITW) 2015, Jerusalem, Israel}, pages = {1--5}, year = {2015} }
-
Parallel Coordinate Descent Newton for Efficient L1-Regularized Minimization
Technical report 2013.@article{bian2013parallel, title={Parallel Coordinate Descent Newton Method for Efficient L1-Regularized Minimization}, author={Bian, An and Li, Xiong and Liu, Yuncai and Yang, Ming-Hsuan}, journal={arXiv preprint arXiv:1306.4080}, year={2013} }
-
Bundle CDN: A Highly Parallelized Approach for Large-scale L1-regularized Logistic Regression
ECML 2013.@inproceedings{ecmlBian13, author = {Yatao Bian and Xiong Li and Mingqi Cao and Yuncai Liu}, title = {Bundle CDN: A Highly Parallelized Approach for Large-Scale L1-Regularized Logistic Regression}, booktitle = {ECML/PKDD}, year = {2013}, pages = {81-95} }
-
Parallelized Annealed Particle Filter for Real-Time
Marker-Less Motion Tracking Via Heterogeneous Computing
ICPR 2012.@inproceedings{icprbian12, author = {Yatao Bian and Xu Zhao and Jian Song and Yuncai Liu}, title = {Parallelized Annealed Particle Filter for real-time marker-less motion tracking via heterogeneous computing}, booktitle = {ICPR}, year = {2012}, pages = {2444-2447} }
-
Digitize Your Body and Action in 3-D at Over 10 FPS: Real Time Dense
Voxel Reconstruction and Marker-less Motion
Tracking via GPU Acceleration
Champion technical report of AMD China Accelerated Computing Contest, 2011 [demo].
@article{songbian2013digitize, title={Digitize Your Body and Action in 3-D at Over 10 FPS: Real Time Dense Voxel Reconstruction and Marker-less Motion Tracking via GPU Acceleration}, author={Song, Jian and Bian, Yatao and Yan, Junchi and Zhao, Xu and Liu, Yuncai}, journal={arXiv preprint arXiv:1311.6811}, year={2013} }
Research Projects (to be updated)
Ongoing Projects:
◾ (Graph) Out-of-distribution (OOD) learning in drug AI
The problem of distribution shift is prevalent in various tasks of AI-aided drug discovery. For example, for the task of structure-based virtual screening, the models are often trained on data of known protein targets but have to be tested on unknown targets. Meanwhile, the current model backbone of Drug AI is the graph neural networks (GNNs).
We have built an OOD Dataset Curator and Benchmark for AI-aided Drug Discovery, for details please refer to the project page.
The main research directions include, but not limited to the following:
- Design OOD learning algorithms and theory, such as algorithms for domain generalization and domain adaptation scenarios, so that Drug AI algorithms could work efficiently in the scenarios of distribution shift.
- The combination of OOD learning and deep graph learning. On one hand, one could improve the generalization ability of deep graph learning algorithms in the OOD scenarios (graph OOD learning); on the other hand, one could utilize the strong modeling capabilities to design better OOD algorithms.
The problem of distribution shift is prevalent in various tasks of AI-aided drug discovery. For example, for the task of structure-based virtual screening, the models are often trained on data of known protein targets but have to be tested on unknown targets. Meanwhile, the current model backbone of Drug AI is the graph neural networks (GNNs).
We have built an OOD Dataset Curator and Benchmark for AI-aided Drug Discovery, for details please refer to the project page.
The main research directions include, but not limited to the following:
- Design OOD learning algorithms and theory, such as algorithms for domain generalization and domain adaptation scenarios, so that Drug AI algorithms could work efficiently in the scenarios of distribution shift.
- The combination of OOD learning and deep graph learning. On one hand, one could improve the generalization ability of deep graph learning algorithms in the OOD scenarios (graph OOD learning); on the other hand, one could utilize the strong modeling capabilities to design better OOD algorithms.
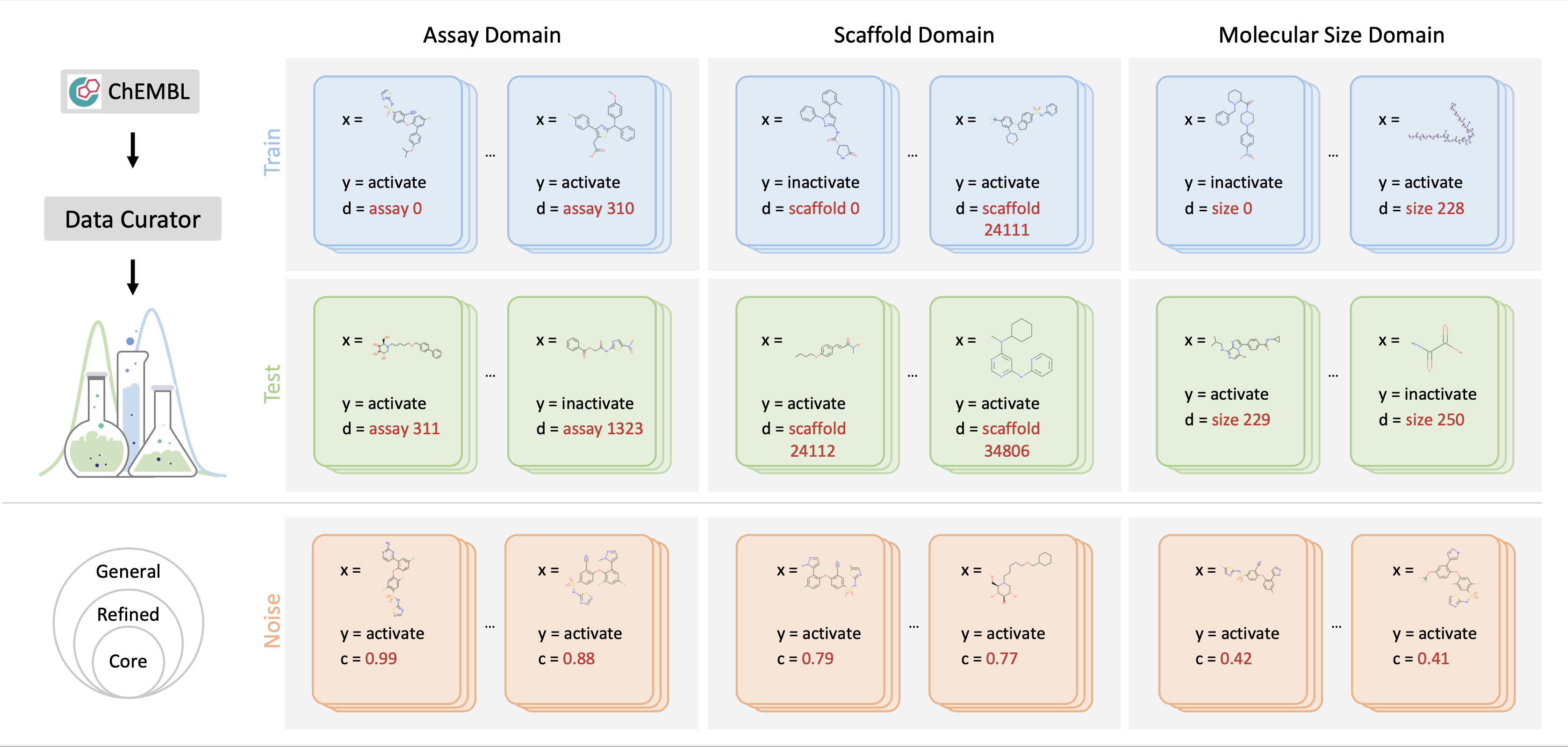
◾ Neural set function learning
Parameterize generic set functions using neural backbones (such as DeepSet-style models and GNN-style models). Develop principled algorithms and theory for set function learning.
For a recent paper, please see Learning Neural Set Functions Under the Optimal Subset Oracle. Here is the project page
Parameterize generic set functions using neural backbones (such as DeepSet-style models and GNN-style models). Develop principled algorithms and theory for set function learning.
For a recent paper, please see Learning Neural Set Functions Under the Optimal Subset Oracle. Here is the project page
Previous Projects:
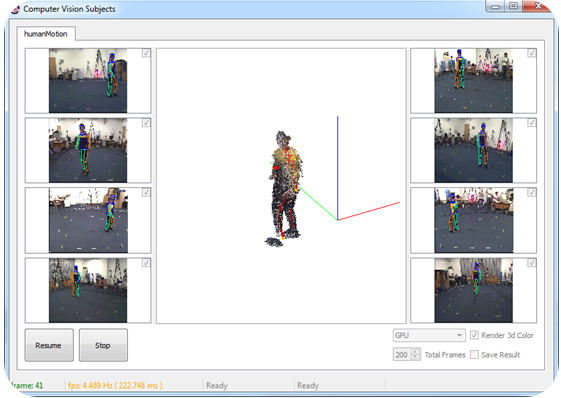
In charge of PAPF design, GPU implementation and optimization.
Proposed Parallelized Annealed Particle Filter (PAPF) algorithm via heterogeneous computing and built a real-time marker-less motion tracking system. Achieved 399 times speedup.
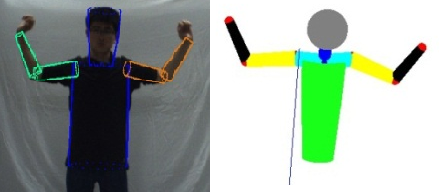
Team leader. Algorithm design, implementation and optimization.
Conducted human upper body pose estimation via generative algorithms . Incorporating generative models and discriminative algorithms to pursue better performance.

Links
(Non-)Convex Optimization
Machine Learning
- Machine Learning (Theory) by John Langford
- The blog of Moritz Hardt
- Probabilistic numerics
- The blog of Alex Smola
- Machine learning summer schools
MISC
- eth-gethired.ch: The job platform for talent made in Switzerland!